About Machine Learning: A Comprehensive Insight into Its Impacts on Business
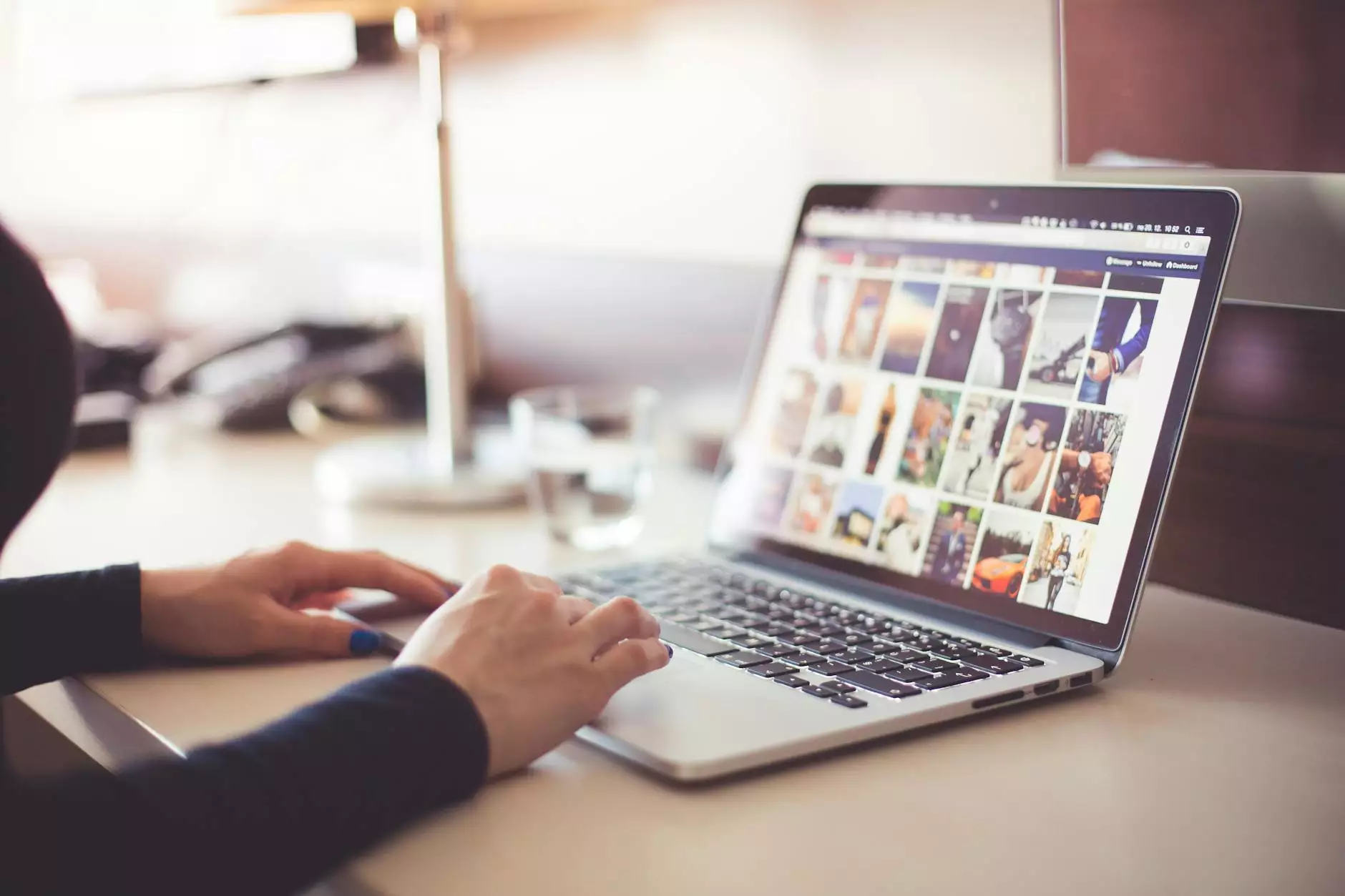
Machine Learning (ML) is a subset of artificial intelligence (AI) that empowers systems to learn and improve from experience without explicit programming. In today's rapidly evolving digital landscape, understanding about machine learning and its applications is vital for businesses seeking to leverage data for prowess and growth. This article delves into the intricacies of machine learning, its benefits, and how it can reshape the future of organizations across various sectors.
1. The Fundamentals of Machine Learning
At its core, machine learning focuses on the development of algorithms that can analyze data, learn from it, and make informed decisions. Unlike traditional programming where explicit instructions dictate outcomes, ML systems adapt their behavior based on patterns and insights gleaned from the data they process. Here are the key components of machine learning:
- Data: The foundational element of any ML application, data can be structured or unstructured and is critical for training models.
- Algorithms: The set of rules or calculations that the machine learning models use to identify patterns and make predictions.
- Model Training: The process through which an ML algorithm learns from data; this can involve supervised or unsupervised learning methods.
- Prediction: The output generated by the trained model when it processes new data, allowing businesses to forecast trends and behavior.
2. Types of Machine Learning
Machine learning can be categorized into three primary types, each with its own unique applications and benefits:
2.1 Supervised Learning
In supervised learning, the model is trained on labeled data, meaning that the input data is paired with the correct output. The model learns to map inputs to outputs and can make predictions on unseen data. Common applications include:
- Fraud detection in financial transactions
- Email spam filtering
- Predictive analytics in customer behavior
2.2 Unsupervised Learning
Unsupervised learning works with unlabeled data, where the algorithm identifies patterns and relationships in the data without prior knowledge of outcomes. This type is useful for:
- Customer segmentation for targeted marketing
- Identifying anomalies for fraud prevention
- Market basket analysis in retail
2.3 Reinforcement Learning
Reinforcement learning is a type of ML where agents learn to make decisions based on rewards and penalties. It is particularly effective in complex environments where sequential decision-making is crucial. Applications include:
- Robotics for navigation and manipulation
- Game playing, such as competitive online gaming
- Autonomous vehicles making real-time driving decisions
3. The Value of Machine Learning in Business
Incorporating machine learning into business operations can yield significant benefits, improving efficiency, productivity, and customer satisfaction. Below are key areas where ML excels:
3.1 Enhanced Decision-Making
Machine learning enables data-driven decision-making, allowing businesses to analyze vast amounts of data and draw actionable insights. By utilizing predictive analytics, companies can anticipate market trends, customer needs, and potential risks, thereby making informed strategic decisions.
3.2 Increase in Operational Efficiency
Automation powered by machine learning can streamline processes, reduce manual efforts, and minimize errors. ML can optimize supply chain logistics, manage inventory levels, and improve manufacturing processes to lower costs significantly while maintaining quality.
3.3 Improved Customer Experience
Machine learning algorithms can enhance customer engagement through personalized experiences. Businesses can analyze customer behavior, preferences, and feedback to tailor services and recommendations, leading to higher satisfaction and loyalty.
3.4 Fraud Detection and Risk Management
Financial institutions leverage machine learning to identify fraudulent transactions and reduce losses. By continuously scanning transaction patterns, ML identifies anomalies that may indicate fraud, thereby safeguarding assets and enhancing trust.
4. Implementing Machine Learning in Business
Transitioning to a machine-learning-driven approach involves a strategic roadmap that includes:
4.1 Defining Objectives
Establish clear objectives for what the business aims to achieve with machine learning. Identify specific problems that need addressing and the subsequent goals that the ML initiative should reach.
4.2 Data Collection and Preparation
Success in machine learning relies heavily on the quality of data. Businesses must gather relevant data, clean it, and ensure it is structured appropriately for analysis. Data gathering can be achieved through:
- Customer interactions and feedback
- Transaction records
- Market research insights
4.3 Model Selection and Training
Choosing the right machine learning model is critical. The model must align with business goals and the type of data available. Experimenting with various algorithms and tuning hyperparameters is essential for achieving optimal performance. Consistent testing and validation ensure reliability.
4.4 Integration and Deployment
Once a model is trained and validated, it needs to be integrated into existing systems. This deployment phase includes ensuring the model can process new data efficiently and provide actionable insights to stakeholders.
5. The Future of Machine Learning in Business
As technology advances, the landscape of machine learning will continue to evolve, leading to unprecedented transformations in business operations. Future trends include:
- More Semantic Understanding: Machines will increasingly understand not just data but context, leading to more natural interactions with users.
- Greater Augmented Intelligence: Combining human intuition with machine learning capabilities will enhance decision-making processes and creative solutions.
- Real-Time Analytics: The ability to analyze data as it streams will provide immediate insights and allow for quicker reactions in dynamic market conditions.
6. Conclusion
Understanding about machine learning is crucial as businesses strive for growth and innovation in an increasingly data-driven world. By embracing machine learning, organizations can enhance their decision-making processes, optimize operations, improve customer satisfaction, and stay ahead of the competition. The journey toward incorporating machine learning may be challenging, but the benefits it offers are transformative and far-reaching, paving the way for a smarter and more efficient business landscape.